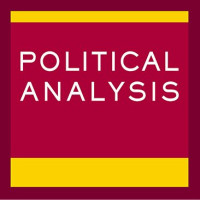
Miklós Sebők and Zoltán Kacsuk have published an article entitled ’The Multiclass Classification of Newspaper Articles with Machine Learning: The Hybrid Binary Snowball Approach’ in Political Analysis.
Short summary:
The article presents a machine learning-based solution for matching the performance of the gold standard of double-blind human coding when it comes to content analysis in comparative politics. The authors combine a quantitative text analysis approach with supervised learning and limited human resources in order to classify the front-page articles of a leading Hungarian daily newspaper based on their full text. The classification of the imbalanced classes of topics is handled by a hybrid binary snowball workflow. This relies on limited human resources as well as supervised learning; it simplifies the multiclass problem to one of binary choice; and it is based on a snowball approach as the authors augment the training set with machine-classified observations after each successful round and also between corpora. The results show that this approach provided better precision results than what is customary for human coders and most computer-assisted coding projects.
Follow this link for the ‘open access’ article.
Sebők, M. and Kacsuk, Z. (2020) “The Multiclass Classification of Newspaper Articles with Machine Learning: The Hybrid Binary Snowball Approach,” Political Analysis. Cambridge University Press, pp. 1–14. doi: 10.1017/pan.2020.27.